- Start
- 1. Regressionsanalyse mit Regression Online
-
2. Data Analysis with Linear Regression Calculator
- Benefit 1: Interpretable Data Analysis
- Benefit 2: Pattern Recognition through Regression Analysis
- Benefit 3: Efficient Computation
- Benefit 4: Foundation Model for Deep Learning and ML
- Benefit 5: Supporting Decisions through Regression Analysis
- Benefit 6: Exploratory Data Analysis
- Benefit 7: Versatile Data Analysis
Linear regression is a statistical method used to understand the relationship between
two or more things. It helps us to make predictions about what might happen in the future
based on what has happened in the past.
For example, if we want to know how the price of a property relates to its size, we can use
simple linear regression. We would collect information about a group of properties and their
price and size. Then we would use a mathematical formula to find the best line that represents
the relationship between price and size. With this information, we can make predictions about
the price of a property based on its size.
Linear regression can also be used to understand relationships between more than two things.
For example, it can be used to understand the relationship between a person's education level,
their work experience and their salary.
verstehen.
The goal of linear regression is to find the line that best fits the data and helps us make
accurate predictions. It can be used in many different fields, such as economics, psychology and
biology, to understand complex relationships and make decisions based on the information we have.
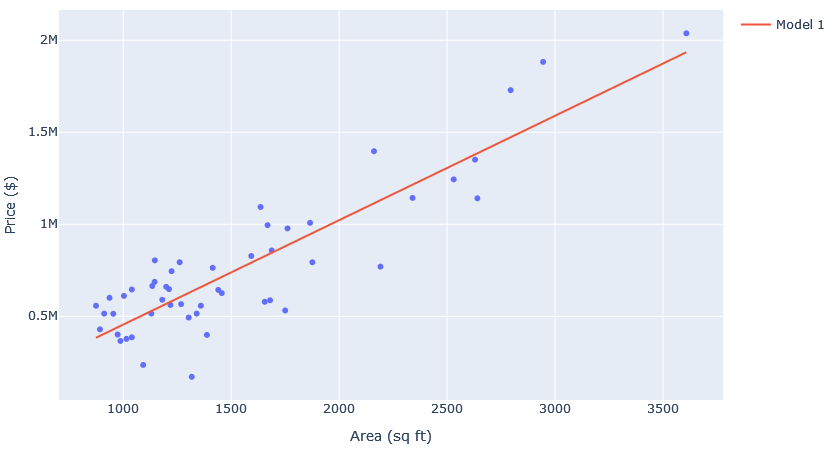
Regression Analysis with Regression Online
Regression Online offers numerous advantages with its online calculator for linear regression, especially for users who want to perform quick and precise analyses without in-depth knowledge of statistical programming or complex software. One of the main reasons for using our online calculator is its ease of use. Regression Online is intuitively designed and allows users to easily upload data and get instant results. This saves time and reduces the effort associated with installing and learning extensive software packages. In addition, the online linear regression calculator provides a visual representation of the results, including regression lines and scatter plots, making it easier to understand and interpret the data. Regression Online is therefore also ideal for ad hoc analyses and an aid to support decision-making.
Another advantage is accessibility: Regression Online can be accessed from any device with internet access, which increases flexibility and allows analyses to be carried out regardless of location. In addition, Regression Online is free or inexpensive in the Pro version, making it an attractive option for students, small businesses and individuals who do not have extensive resources for expensive software solutions. In addition, Regression Online also supports the export of results in various formats, which facilitates further processing and integration into reports or presentations. Overall, Regression Online for linear regression is a practical, efficient and accessible solution for performing statistical analysis.
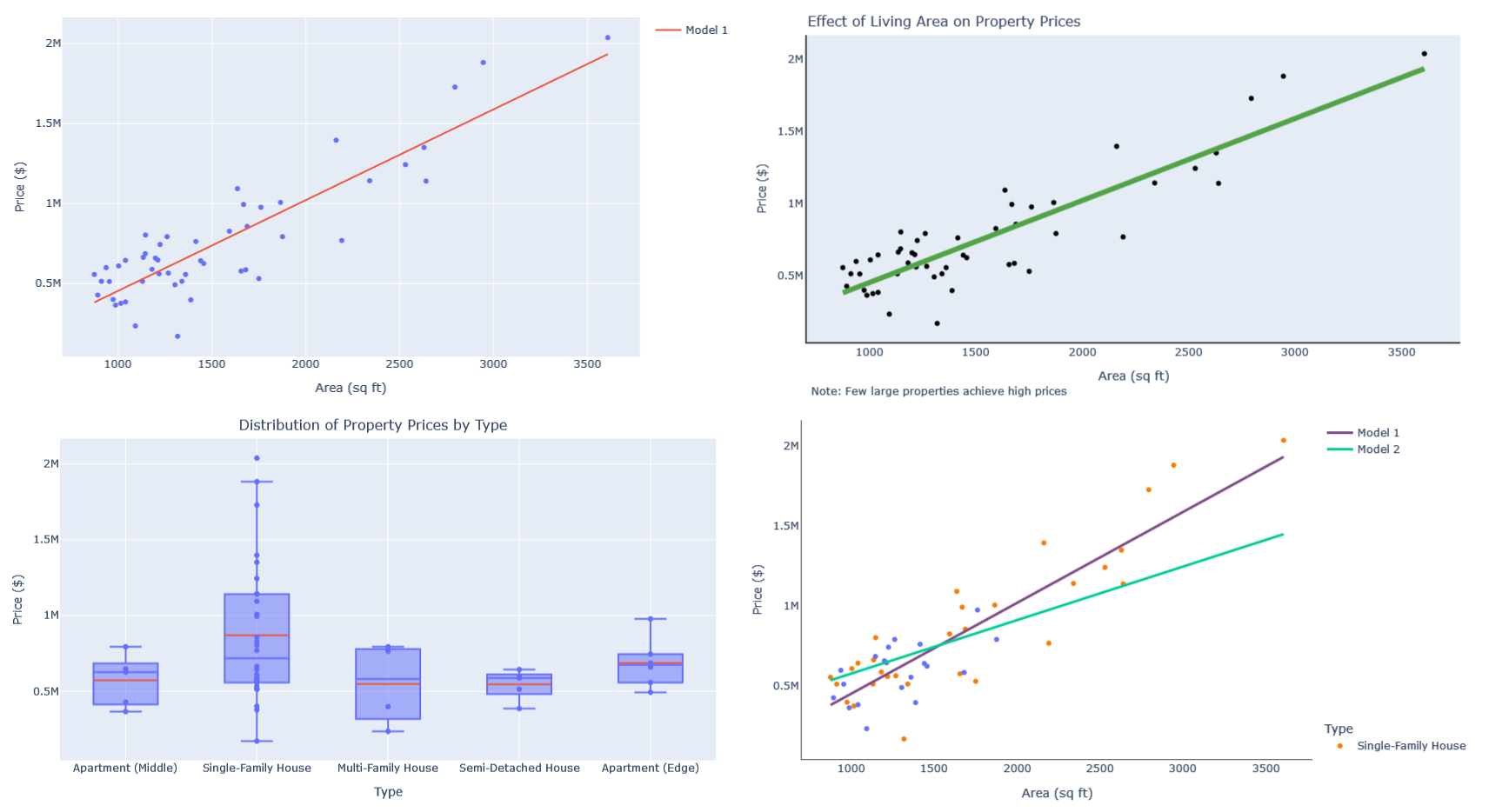
Features of Regression Online
- Dynamic selection of relevant variables for the analysis
- Upload your own data via drag and drop
- Interactive table available for immediate editing
- Responsive plots for data exploration and data analysis
- Various styling options available for plots
- Detailed results of the regression analysis available for download
Data Analysis with Linear Regression Calculator
Linear regression is a fundamental method of data analysis that is widely used in the quantitative sciences and in the field of data science. Linear regression enables scientists to make predictions, test hypotheses and quantify relationships between variables. For analysts and entrepreneurs, linear regression provides a way to recognize patterns in their data to make better decisions.
In addition, linear regression is flexible enough to form a basis for more complex models where multiple independent variables are considered. In science and business, linear regression is an indispensable tool for analyzing and interpreting data, as it provides insights into causal relationships and influencing factors. The advantages of the regression model are
Interpretable Data Analysis
The interpretability of linear regression is a major advantage of this statistical method and contributes significantly to its widespread use in various scientific disciplines. A key feature of linear regression is its simplicity and transparency. The equation provides a clear and understandable representation of the relationship between the dependent variable Y and the independent variable X. The intercept indicates the value of Y when X is zero, while the slope describes the change in Y when X increases by one unit. These parameters are directly interpretable and allow analysts to quantify the strength and direction of the relationship between the variables.
Moreover, linear regression allows the calculation of confidence intervals and p-values, which provide information on the statistical significance of the estimated parameters. These statistical measures help to assess the reliability of the results and to draw well-founded conclusions. Another advantage is the ability to use residual plots and other diagnostic tools to check the suitability of the model and potential violations of assumptions. Despite its simplicity, linear regression thus offers high explanatory and predictive power, making it an indispensable tool in data analysis.
Pattern Recognition through Regression Analysis
Today, linear regression is used in many areas of data analysis and machine learning. By using regression models, researchers and analysts can identify patterns in large data sets and use them to predict future values. Linear regression models the relationship between a dependent variable and one or more independent variables by drawing a line through the data points that provides the best estimates of the dependent variable. This method is particularly useful for identifying linear relationships and trends in the data. One example is the analysis of sales data, where sales are examined in relation to advertising expenditure. Here, linear regression can help to understand the pattern of sales figures and predict future sales based on planned advertising measures.
Furthermore, linear regression enables the identification of outliers and unusual patterns that could indicate anomalies or special events. In practice, advanced techniques such as multivariate linear regression are often used to identify more complex patterns involving multiple independent variables.
Efficient Computation
The efficiency of linear regression is one of the main reasons for its widespread use in data analysis and machine learning. One of the greatest strengths of this method lies in its computational simplicity and comparatively low complexity. The calculation of the regression coefficients essentially requires the solution of a linear system of equations, which can be performed extremely quickly and resource-efficiently with modern computers, even with large data sets. This efficiency makes it possible to apply linear regression to a wide range of problems, from predicting share prices to analyzing market research data and evaluating scientific experiments.
Additionally, linear regression requires less memory and less computing time compared to more complex models, which makes it particularly useful for real-time applications. Another advantage is the robustness of the method against multicollinearity and the existence of good procedures for model evaluation, such as the coefficient of determination R² and the analysis of residuals. These properties make it possible to quickly and efficiently create valid models that provide reliable predictions. To summarize, linear regression is an extremely valuable method in modern data analysis due to its computational efficiency, simplicity and robustness.
Foundation Model for Deep Learning and ML
Linear regression often serves as a foundation and basic model in the field of machine learning and deep learning. Despite the simplicity of this method, it provides a clear and understandable foundation on which more complex models can be built. Linear regression is often the first step in modelling as it illustrates basic concepts such as minimizing errors and optimizing parameters. These principles are essential for understanding and developing more advanced models. In machine learning processes, linear regression serves as a benchmark to evaluate the performance of new, more complex models. If a model based on linear regression delivers satisfactory results, you can be sure that it is a solid starting point for further analysis and model development.
Linear regression is also often used for feature selection and for interpreting the meaning of individual features, which is particularly important when working with high-dimensional data. In deep learning scenarios, linear regression can be used as a loss function or as an activation function in neural networks, especially in the regression of continuous values. Even though neural networks and other more complex models tend to be more powerful, understanding and applying linear regression is essential as it is a simple and interpretable model that provides insights into the data structure and relationships between variables. Thus, linear regression remains an indispensable tool and a starting point for the development and validation of more advanced machine learning models.
Supporting Decisions through Regression Analysis
Companies can use linear regression to support their decision-making processes and thus make better business decisions. As a result, regression analytics offers significant benefits for companies looking to implement data-driven strategies. By applying this data analysis, companies can make accurate predictions and informed decisions based on historical data and trends. For example, sales figures can be analyzed in relation to advertising expenditure, pricing or seasonal influences in order to identify the most effective marketing strategies.
Linear regression enables the identification of key variables that have the greatest impact on business performance, facilitating targeted resource allocation and strategic planning. Another advantage is the ability to perform scenario analyses to simulate potential outcomes under different assumptions, thus minimizing risks and making better use of opportunities. By integrating linear regression into business analyses, companies can achieve greater accuracy in forecasting future developments, leading to improved competitiveness and long-term success.
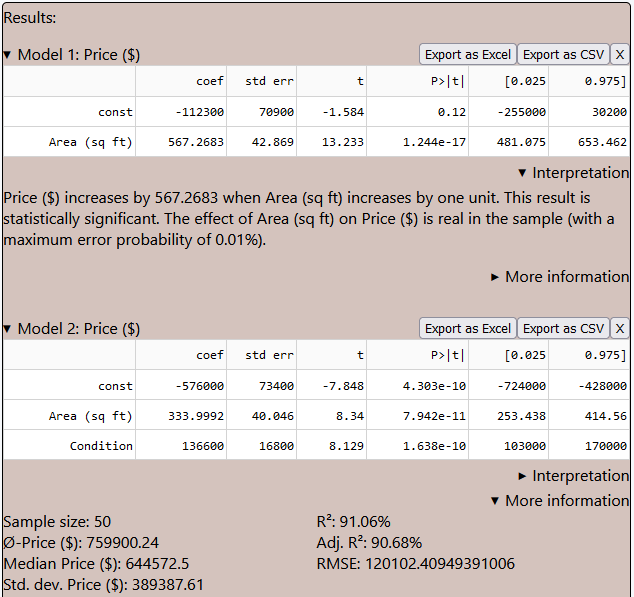
Exploratory Data Analysis
Linear regression plays a crucial role in exploratory data analysis as it helps to uncover and understand patterns and relationships in the data. Exploratory data analysis is about initially identifying unknown structures and relationships within a dataset, and linear regression is a valuable tool for this. By creating a linear model, analysts can quantify the nature of the relationship between different variables by fitting a straight line to the data that best approximates the observed values. This makes it possible to identify both positive and negative correlations and assess whether and to what extent an independent variable influences a dependent variable.
The regression model also helps to identify outliers and unusual observations that may indicate special conditions or errors. In addition, by analyzing the regression coefficients and statistical significance, further insight can be gained into which variables actually have a significant impact on the result. These findings are particularly valuable for formulating hypotheses that can be tested in further analyses or experiments. Overall, linear regression in exploratory data analysis helps to gain a deeper understanding of the data, make well-founded assumptions and create the basis for further analytical steps and modeling.
Versatile Data Analysis
The versatility of linear regression makes it an indispensable tool in a variety of scientific and industrial applications. Its simplicity and interpretability make it possible to quickly model and understand basic relationships between variables. This makes linear regression a preferred method in many fields, such as econometrics for predicting economic indicators, biostatistics for analyzing medical data, and environmental science for modeling climate change. In addition, linear regression plays a crucial role in data analysis and decision-making in industry.
Regression Analysis helps companies to create sales forecasts, evaluate the effectiveness of marketing campaigns and optimize operational costs. By analyzing sales data, for example, companies can determine which factors have the greatest impact on sales and take targeted measures to increase sales. In finance, linear regression is used to assess risks and optimize investment portfolios. It also enables the modeling of market trends and the prediction of future developments, which is essential for strategic planning. Overall, linear regression offers broad applicability due to its adaptability and robustness, making it a cornerstone of data analysis and modeling.