- Start
- Use case 1: Analyzing the Impact of Advertising Channels on Revenue
- Use case 2: Investigating Optimal Pricing Points
- Use case 3: Using Sales Proposals to Estimate Future Sales
- Use case 4: Identifying and Understanding Your Customer Base
- Use case 5: How Product Features Affect Sales and Pricing
Revenue analysis is a critical function for any business seeking to optimize growth, profitability, and strategic decision-making. One of the most effective ways to uncover revenue trends, forecast future performance, and identify key drivers of success is through data-driven insights. Linear regression, a widely used analytical approach, enables businesses to establish relationships between revenue and influencing factors such as pricing, marketing spend, customer demographics, and market conditions. By leveraging this method, business leaders can make more informed decisions, allocate resources efficiently, and drive sustainable revenue growth. In this article, we will explore how linear regression can be applied to revenue analysis and how it can enhance strategic planning.
Understanding the potential of linear regression in revenue analysis is just the first step. To truly appreciate its impact, it’s essential to explore how businesses can apply it in real-world scenarios. From optimizing pricing strategies to predicting future sales trends, linear regression offers valuable insights that drive data-backed decision-making. In the next section, we will delve into specific use cases where this approach can help businesses enhance revenue forecasting, improve customer segmentation, and maximize profitability.
Analyzing the Impact of Advertising Channels on Revenue
In today's competitive market, businesses invest heavily in various advertising channels: digital, print, TV, and social media - expecting a strong return on investment. However, understanding which channels contribute the most to revenue can be challenging. By using data-driven insights, companies can evaluate the effectiveness of each advertising source and allocate budgets more efficiently. A structured approach to analyzing historical data helps businesses identify trends, optimize marketing spend, and maximize revenue growth. For example:
The scatter plots above illustrate the relationship between weekly sales and advertising budgets across three key channels: TV, Social Media, and Internet. Each plot includes a trend line representing the correlation between ad spending and sales, providing valuable insights into how each channel influences revenue. Note the varying scales on the x-axis!!
-
TV Advertising: Positive Impact
The first analysis shows a positive correlation between TV ad spend and weekly sales. As companies increase their TV advertising budget, sales tend to rise consistently. The relatively tight clustering of data points around the trend line suggests a high predictive power, meaning businesses can reliably estimate revenue growth based on their TV advertising spend. This indicates that TV remains a powerful and effective advertising medium, particularly for older consumer markets. -
Social Media Advertising: Stronger Positive Impact but High Variance
The second model illustrates the relationship between social media ad spend and sales. While there is a very strong positive correlation, the data points exhibit greater spread around the trend line compared to TV. This suggests that while social media advertising contributes a lot to revenue growth, its effectiveness is less predictable. Other factors, such as target audience engagement, ad quality, or platform algorithms, may play a role in determining its impact. While social media advertising is effective, businesses may need to fine-tune their strategies! -
Internet Advertising: Medium Strong Positive Impact and Dispersion
The third chart presents a strong correlation between Internet ad spending and sales too. While the trend line slopes upward, the data points are also more dispersed, indicating a less consistent impact. This suggests that internet advertising alone may not be a reliable driver of revenue growth, possibly due to factors like banner ad fatigue, ad-blocker usage, or ineffective targeting. Businesses investing in digital advertising should consider integrating it with other channels or refining their online ad strategies to improve results.
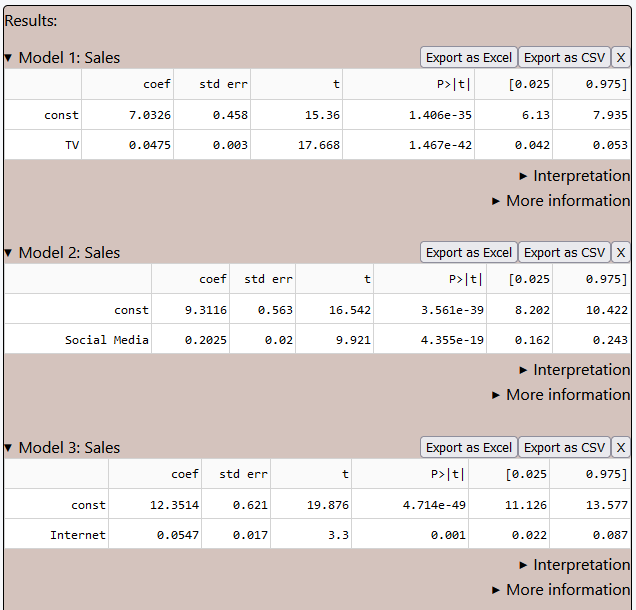
→ TV:
- The coefficient for TV ads (0.0475) tells us that that for every additional dollar spent on TV advertising, sales are expected to increase by only 0.0475 units.
- The confidence interval ([0.162, 0.243]) suggests a reliable range for that coefficient.
- TV advertising has a moderate positive and consistent impact on sales, making it an effective channel among the three.
- The coefficient for Social Media ads (0.2025) indicates that for every additional dollar spent on Social Media advertising, sales are expected to increase by 0.2025 units
- The confidence interval ([0.162, 0.243]) for that coefficient shows the widest range, indicating the most variability in impact.
- Social Media advertising has the highest impact on sales, but its impact is also the most variable
- For every additional dollar spent on Internet advertising, sales increase by 0.0547 units on average.
- The confidence interval ([0.022, 0.087]) suggests a broader range for the coefficient meaning there is some variability in impact.
- Internet advertising has a positive effect on sales but the effect is less consistent than TV advertising.
By allocating marketing budgets strategically based on these insights, businesses can maximize their advertising ROI and drive sustainable revenue growth. Businesses can prioritize TV marketing for slow but predictable revenue growth. Social Media advertising is very effective but might require strategic optimization. Companies should focus on engagement, audience targeting, and content quality to maximize effectiveness. Internet advertising alone also does not guarantee strong sales growth. It may work best as a supporting channel rather than a primary driver of revenue.
Investigating Optimal Pricing Points
Setting the right price is one of the most crucial decisions for any business. Price too high, and customers may turn away; price too low, and profitability suffers. Linear regression helps businesses analyze past sales data to identify the relationship between pricing and revenue, revealing the optimal price point that maximizes earnings. By leveraging historical trends, companies can make data-driven pricing decisions that balance customer demand with profitability, ensuring sustainable growth in competitive markets. Consider this scenario:
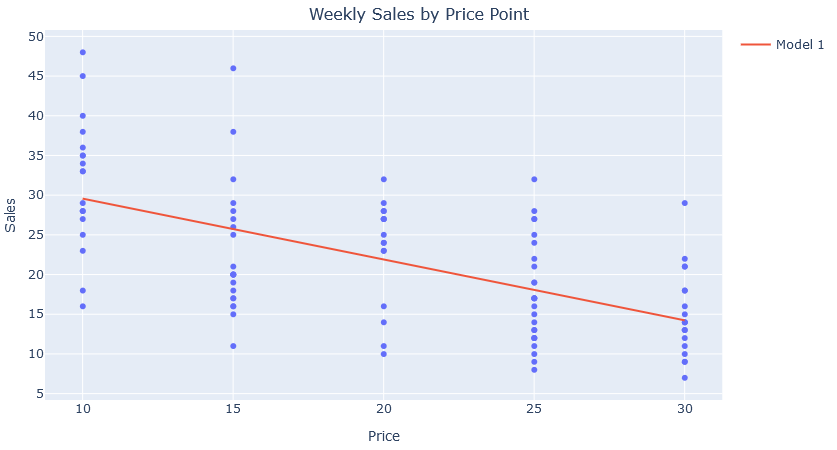
The scatter plot above illustrates the relationship between weekly sales and price points, with a fitted
linear regression line providing insights into the overall trend. As expected, the graph shows a negative
correlation between price and sales: When prices increase, the number of units sold decreases. This is a
common pattern in consumer behavior, where higher costs lead to lower demand. At the lowest price point ($10),
sales volumes are at their highest, reaching nearly 50 units per week in some instances. Conversely, at the
highest price point ($30), sales drop significantly, with many data points clustering below 20 units per week.
The linear regression analysis provides a basis for estimating how sales might respond to further price adjustments.
Businesses can use this insight to identify optimal price points that balance volume and revenue.
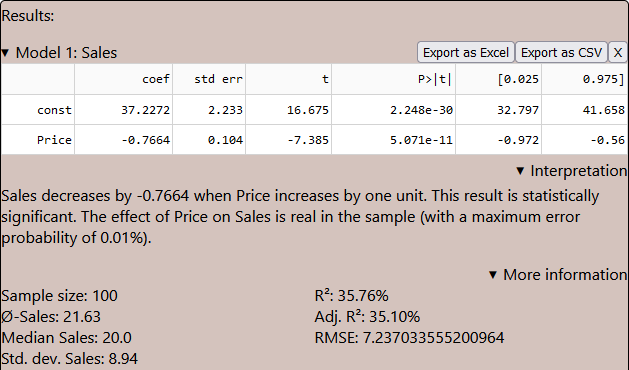
The results of the regression analysis provides quantitative insights into how pricing affects sales. The main takeaways are:
- Relationship Between Price and Sales
- The coefficient for Price is -0.7664, indicating that for every $1 increase in price, sales decrease by approximately 0.77 units on average.
- This negative coefficient confirms the expected inverse relationship between price and demand - higher prices result in lower sales.
-
Statistical Significance
- The p-value for Price (5.071e-11) is extremely small, meaning the impact of price on sales is statistically significant. The decreasing relationship is strong and unlikely due to random chance.
- This analysis confirms that price adjustments significantly impact revenue.
- While price increases may reduce sales volume, businesses need to evaluate whether the higher margins per unit compensate for the drop in quantity sold!
- Given that price explains only 35.10% of sales variation, businesses should consider additional factors like promotional strategies, brand positioning, and competitor pricing to refine their pricing strategy.
Using Sales Proposals to Estimate Future Sales
Sales proposals serve as a strong indicator of upcoming sales, but their actual conversion into revenue remains uncertain. By analyzing historical data on weekly proposals sent and the corresponding sales closed, businesses can establish patterns that help estimate future revenue. This data-driven approach enables companies to allocate resources efficiently, set realistic revenue targets, and optimize sales strategies.
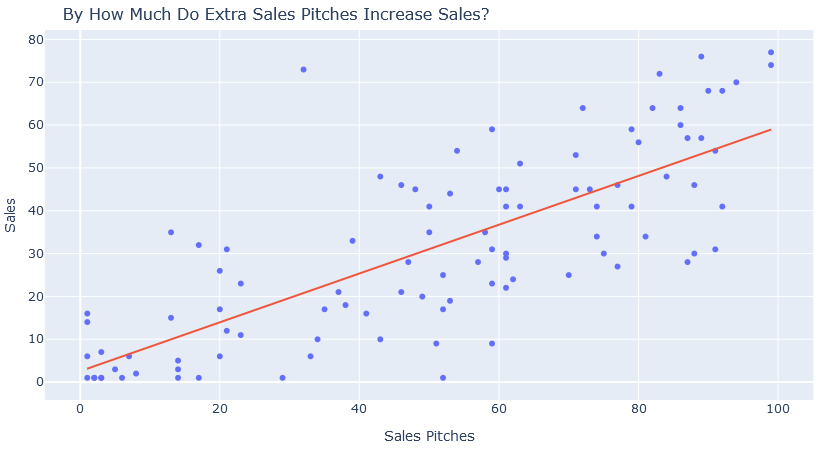
The scatter plot above illustrates the relationship between the number of weekly sales pitches (proposals) and the resulting sales figures. Each blue dot represents a data point from past sales performance, while the red line represents the trend - showing a positive correlation between the number of proposals and sales. The trend line suggests that as businesses increase their number of sales proposals, revenue tends to rise correspondingly. This relationship allows sales leaders to estimate future revenue by analyzing the historical success rate of proposals. If past data indicates a consistent conversion rate, companies can predict revenue more accurately based on the number of proposals expected in a given period.
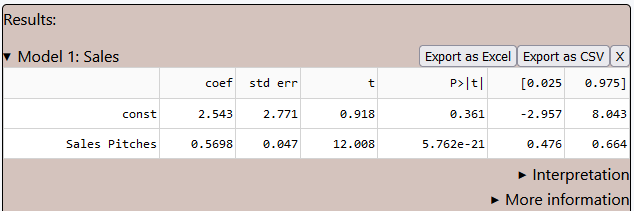
The above table presents the results of a linear regression analysis where weekly sales pitches is the independent variable (predictor) and sales is the dependent variable (outcome). The model helps quantify the impact of additional sales pitches on the number of actual sales. The key findings are as follows:
- Intercept (const) = 2.543
- This is the baseline sales value when no sales pitches are made.
- It suggests that even with zero pitches, an average of 2.54 sales might still occur due to other factors.
- The p-value (0.361) indicates that this coefficient is not statistically significant, meaning the baseline sales might be due to randomness rather than a meaningful trend.
- Sales Pitches Coefficient = 0.5698
- This means that for each additional sales pitch, expected sales increase by approximately 0.57 units.
- In simpler terms, every 10 additional sales pitches result in approximately 5.7 more sales.
- The p-value (5.76e-21) is extremely small, indicating strong statistical significance. This confirms that the relationship between sales pitches and sales is not due to chance.
- The results confirm a strong and statistically significant positive relationship between the number of sales pitches and actual sales and validate the assumption that more sales pitches lead to higher sales.
- Sales teams can use this model to forecast revenue based on the number of proposals they plan to send.
- Since the intercept is not statistically significant, businesses should not rely on a base sales assumption and should instead focus on their outreach efforts.
Identifying and Understanding Your Customer Base
Knowing your customers is the foundation of any successful business strategy. By analyzing past revenue data, businesses can uncover patterns in customer behavior, preferences, and purchasing habits. Linear regression helps identify which factors—such as demographics, seasonality, or marketing efforts - are most closely linked to revenue growth. With these insights, companies can refine their targeting strategies, optimize pricing, and enhance customer engagement to drive sustainable revenue growth.

In this case, the relationship between customer age and shopping cart value, a pattern emerges: an inverse
U-shape. This means that both younger and older customers tend to spend less, while the highest spending comes
from middle-aged customers. Here, they have about 15% more purchasing power! This insight is valuable for
businesses looking to optimize their marketing and pricing strategies.
The analysis suggests that for this exemplary product or service, younger customers may have lower purchasing
power or different spending priorities, while older customers might be more selective in their purchases.
Middle-aged consumers, on the other hand, likely represent the peak of financial stability and spending
capacity, making them the most valuable segment for revenue generation.
This finding has practical implications for businesses. Marketing efforts can be adjusted to appeal more
effectively to younger and older demographics, potentially increasing their average spending. Additionally,
businesses can focus on retaining and expanding their middle-aged customer base, ensuring that their product
offerings and promotional strategies align with the needs and preferences of this high-value segment.
Understanding these spending behaviors allows companies to make data-driven decisions that enhance customer
engagement and maximize revenue potential.
NOW BEWARE: While linear regression is a powerful tool for identifying relationships between variables,
it assumes a straight-line relationship between them. However, as seen in our analysis of shopping cart value
by customer age, the data follows an inverse U-shape rather than a simple upward or downward trend. This means
that a standard linear regression model would struggle to capture the full picture, potentially leading to
misleading conclusions.
In this case, applying a basic linear regression model could either underestimate or overestimate spending
trends. For example, it might suggest that spending simply increases or decreases with age, missing the
crucial peak among middle-aged customers. This happens because linear regression forces a straight-line
relationship, even when the data naturally follows a curve.
Being aware of these limitations is crucial when using linear regression for revenue analysis. By recognizing when
data follows a non-linear trend, businesses can adjust their approach to ensure more accurate insights and better
decision-making.
How Product Characteristics Affect Sales and Pricing
One of the most fundamental ways businesses can use data analysis with linear regression is to understand how
specific product characteristics influence sales and pricing. By analyzing the relationship between a key
product attribute and its impact on revenue, companies can make better pricing decisions, optimize their offerings,
and maximize profitability.
One classic example: the real estate industry. Here, property prices are often influenced by factors
such as location, number of bedrooms, and most notably, square footage. Suppose a real estate firm wants to determine
how the size of a house affects its selling price.
→ By applying linear regression, they can analyze historical sales data and establish a trend between the area
measured in square feet and the final selling price.
→ If the analysis reveals a strong positive correlation, meaning that larger homes generally sell for higher
prices, real estate professionals can use this insight to make strategic pricing decisions. For instance, they
can predict the market value of a newly listed property based on its size
For realtors, it enables them to price homes more accurately based on market data rather than relying solely on intuition or broad comparisons.
This leads to competitive pricing that attracts buyers while maximizing returns for sellers.
Additionally, realtors can use these insights to identify undervalued properties in the market. If a home's price does not align
with its size and market trends, it may present a profitable investment opportunity. Similarly, realtors can provide data-backed
guidance to buyers, helping them assess whether a property is fairly priced or likely to appreciate in value.
Developers can use historical sales data to determine the optimal price per square foot, ensuring that new projects align with market demand and maximize profitability. This helps refining construction strategies for better profitability: Whether it's designing smaller, more affordable units in urban areas or larger homes in suburban markets, linear regression insights enable developers to align their projects with real market demand and adjust their strategies to meet emerging buyer preferences.
By leveraging linear regression to analyze product characteristics, businesses—whether in real estate or other
industries—can make data-driven decisions that align with market trends, enhance pricing strategies, and ultimately
drive higher revenue.In this case, the analysis empowers realtors with data-driven insights that enhance pricing strategies,
improve client advisory services, and create more efficient sales processes—leading to better outcomes for both buyers and sellers.
If you're not in real estate, don't worry - this approach isn't just for property pricing! Understanding how product characteristics influence sales and pricing applies
to almost every industry, from physical products, digital services, to experiences, knowing how key attributes impact pricing can help you make smarter,
data-driven decisions.